Generative AI is revolutionizing the way that content is created as user-friendly tools have made their way into the hands of the masses around the world. The possibilities seem endless, and their application may impact almost every sector of society. Since the beginning of time, though, every novel technology suffused with promise also provides an opportunity for misuse and abuse by those so inclined. But how can we reduce or even avoid victimization? Do standard recommendations related to internet safety and security apply here as well? Are there fresh methods and approaches we should adopt to address these new harms? And what are the responsibilities of the major stakeholders in this conversation?
In my last piece, I explained in detail some manifestations of generative AI harm. Let’s now shift our focus to preventive efforts by platforms and users. To be sure, it is incumbent upon all of us to vigilantly ensure the negatives of generative AI do not outweigh the positives. Below, we provide some guidance and direction on exactly how to do this.
The Role of Online Platforms in Preventing Generative AI Abuse
Social media and gaming companies that provide spaces for online interaction among users should endeavor to get in front of the potential problems that generative AI might facilitate. Otherwise, they will get caught with their proverbial pants down and unwittingly further contribute to the rapid erosion of public confidence and trust in their ability to safeguard their userbase. From my perspective, their contributions will not take a great deal of novel engineering or complex problem-solving. They are, it seems, relatively low-hanging fruit. First and foremost, they must assist in detecting and labeling AI generated content – regardless of whether it is innocuous or potentially harmful. Second, they must continue to educate users in relevant, creative, and highly resonant ways.
Detect and Label AI-Generated Content
Many platforms already use technology which flags unnatural behavior patterns that don’t align with what genuine users typically say and do. This might involve the frequency of posts, the repetition of sentiments or content being shared, whether an account is growing its follower base and social network in inauthentic ways, and whether it interacted with known harassers, deceivers, bots or botnets. These detection systems will continue to learn and adapt from all user-generated content and become better at discerning what is real and what is manipulated, as well as which accounts are genuine and which are fabrications. Companies should continue to devote significant resources to enhancing the accuracy and robustness of these systems immediately.
Detection algorithms can also assist in visually indicating that certain content may be fake through the use of labels. However, an individual who is creating synthetic content via generative AI may not want their creations to be labeled in any way. This is because labels may impose a tremendous burden on the creator (who may have spent a great deal of time working through iterations and determining the best prompts to use), violate their intellectual property rights, and “out” them or otherwise undermine their privacy. I can empathize with all of these concerns.
While it is beyond the scope of this piece to philosophically delve into the pros and cons of labeling AI-generated works, it seems most responsible at this early stage in the generative AI lifecycle to directly help users know exactly what they are seeing. For instance, the use of watermarks or digital signatures embedded within each creation by the generative AI model itself can allow an OS or platform to automatically mark it as such. Perhaps it has a “promo” logo on it (indicating the name of the software tool used). Perhaps AI-generated text is made visually distinct from human-generated text via differences in font, font decoration, or background color. As many of us have seen first-hand, Twitter labels certain content as “misleading information,” “disputed claim” or “unverified claim” while Instagram labels it as “false information” in a way that stands out. This thereby helps the userbase understand the authenticity of what is posted – perhaps especially when displayed in the middle of the media content itself, or prior to it being loaded – so that the user doesn’t miss the warning and grasp the questionable nature of the information.
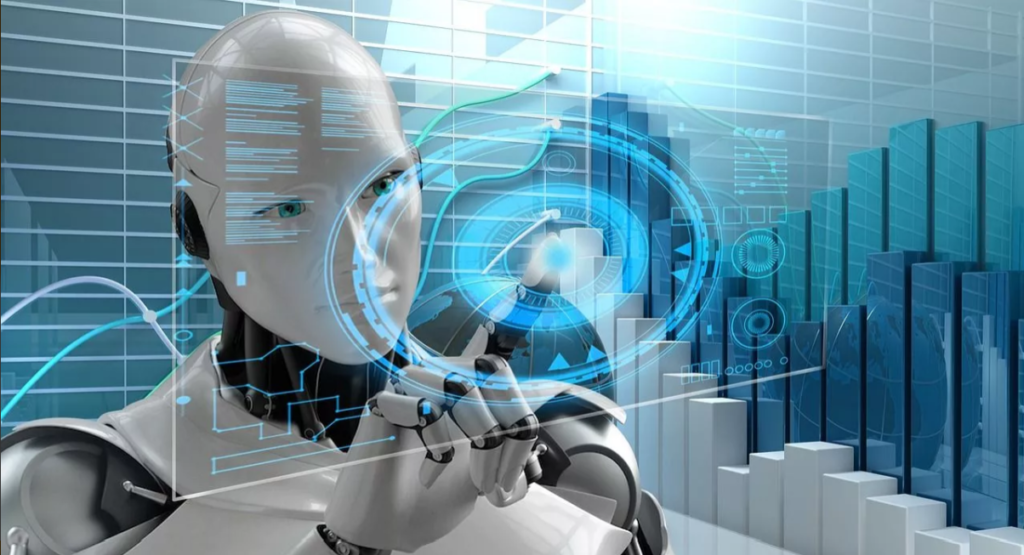
As another idea, images and videos created with the device’s camera and/or within certain apps like Instagram, TikTok, and Snap should be embedded with metadata that stores those variables. At the same time, information about its provenance could be stored on a secure digital ledger system (e.g., blockchain). This would allow individuals to determine if the content in question has been manipulated since the moment it was originally created.
Indeed, labels, watermarks, and annotations are being considered not just with image content, but also with text. Open AI is experimenting with the intentional insertion of certain words within the output of ChatGPT that would clearly identify its origin. Chatbots and related conversational agents, if not identified as such at the outset, would thereby reveal their identity in what they end up saying because of how certain words are included and distributed across sentences. To fight the good fight against harms facilitated via AI-generated content, these techniques provide critical transparency to distinguish what is human-generated from what is AI-generated. Related technologies are under development by researchers in this space, and formal evaluations will help inform norms, laws, policies, and product design as we all move forward.
Provide Anonymized Incidents for Data Cleansing Purposes
Apart from labeling content, platforms should increasingly refine their ability to detect harassment, deepfakes, hate speech, catfishing, and grooming. We understand that new content generated by AI is going to mirror many of the characteristics of the data it is trained upon. In the interest of shared oversight and for accountability purposes, it might be valuable for platforms to submit digital evidence of clear, prohibited incidents to OpenAI, Stability AI, Google, Microsoft, and similar companies so that their training models intentionally remove such content from their large language models (LLMs). This incident data would have to be anonymized, confidentiality agreements and partnerships established, and perhaps governmental mandates required, but this may be one way to keep generative AI models from unwittingly serving as a vector of harassment and harm. As an aside, I just found out that Adobe Firefly’s new generative AI technology allows creators to attach a “Do Not Train” flag to their content as a way to alert models that they should not include it in their training data (h/t to my friend and colleague Nathan Freitas). The concrete incidents of generative AI misuse and abuse that has previously occurred on platforms can and should be similarly tagged.
Verify Genuine Users
For the most part, bots reduce the quality of our experience on social media platforms by not contributing anything meaningful or helpful to the interactions that take place. Moreover, companies like Meta intentionally seek out and delete millions of fake accounts because of the questionable or false information they share. It seems prudent, then, to implement methods to verify that users are legitimate and not automated conversational agents intended to annoy, harass, threaten, deceive, or otherwise detract from a positive online experience. Declaring that artificial engagement and botting are “damaging to the community as a whole,” Twitch has seen success using email and/or phone verification to ensure that channel subscribers and viewers are real people, and this approach can be easily deployed across most account-based apps and sites. Frankly, it should already be an established, regular practice. We’ve recently seen Twitter engage in a mass-deletion of bots across its site; it is curious why this wasn’t done before except to inflate metrics, feign organic growth, and remain relevant as a “major” player in the space. Two-factor or identity document verification are other options to consider to distinguish human users from bots, but these security steps are an additional burden which will get annoying if not elegantly implemented.
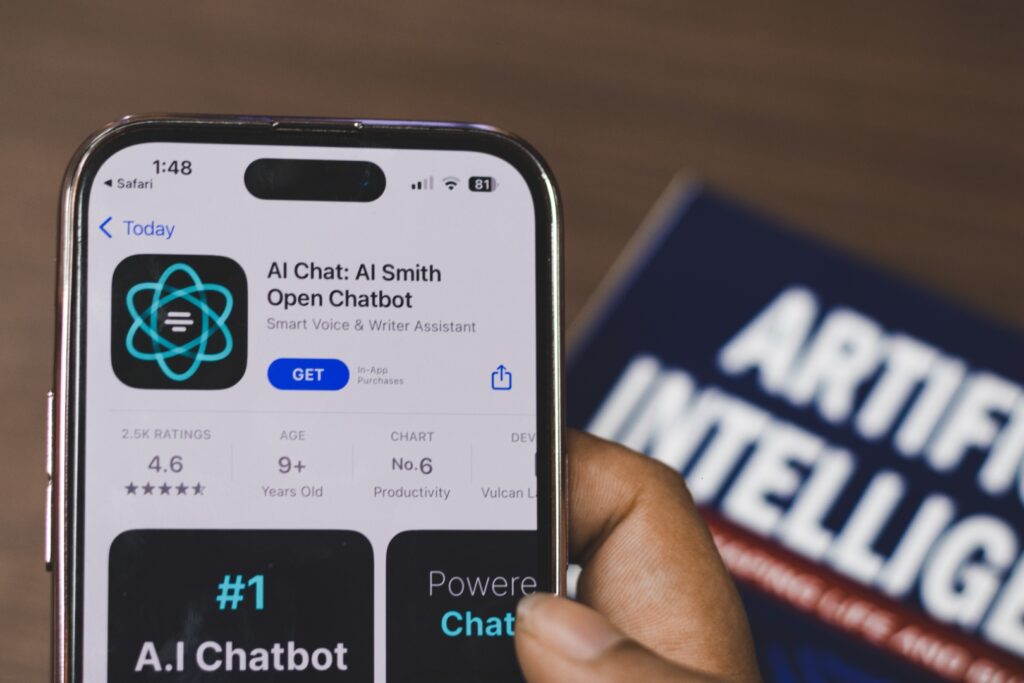
Restrict Generative AI Input, Output, and Training Data
Platforms have, over the years, built internal knowledgebases related to offensive cultural terms and imagery, overt and covert harassment, and coded language use. Ideally, this information might be offered to the developers of major generative AI tools (with oversight, accountability, and legal agreements) so that better restrictions are set in place (e.g., prohibited keywords, the disallowance of sexual content). For instance, Dall-E 2 inhibits the ability for users to make certain keyword requests, scans the output before presenting it, and attempts to minimize the number of nude images in its training data. Midjourney also blocks the use of certain keywords and utilizes some level of human moderation. Stable Diffusion recently stopped using pornographic images in its training data, but there are workarounds.
As we remember that the output created by these tools is predominantly dependent upon the corpus of content analyzed and learned from – as well as the prompts inputted by the user – such restrictions can prevent obvious and blatant misuse. More subtle or insidious modalities of harassment and harm might still be generated, but at least they can be still flagged as questionable and then sent to a human moderator for manual review and confirmation.
As a follow up, content moderators have a significant role to play in this approach, and underscore the importance of humans in the loop no matter what aspect of AI we are discussing. As one example, OpenAI used human reviewers to identify highly violent and sexual content within its training data for DALL-E 2. Then, they subsequently built a new, smaller model used to detect such content in its large model. The technology could then be used to detect whether to allow or deny new borderline violent or sexual images from users, given that humans had created and agreed on a comparative baseline of content deemed too violent or too sexual. It stands to reason that this same practice can be useful with all types of content – text, image, audio, video, and on the whole help mitigate this particular risk of generative AI models.
Educate More Seamlessly Within the User Experience
We – and others in the field of online safety – have pounded the table for more educational programming and resources from social media and gaming platforms to help users level up in knowledge, skills, and abilities to protect themselves online and optimize their environment for healthy, enjoyable interactions. As a result, most platforms have built “Safety Hubs” or “Help Centers” designed to hold the hands of users in setting up app-specific controls through clear descriptions, screenshots, and walkthroughs. Advancing from those initiatives, Roblox designed a Civility curriculum, Google built a virtual world called “Interland,” and TikTok launched campaigns to share strategies and safety features via in-person workshops in the community.
While it’s hard to measure the true benefits of such efforts, each still requires the user to specifically go to a site, page, web property, subsection of the app, or local event in order to learn the proper techniques and steps. If this is not mandated by a school or workplace, the reality is that the vast majority of people won’t go to, or use, these resources until they are in a situation that demands it. That is, they won’t until they are victimized – which the resources were largely built to prevent in the first place. Accordingly, even though “education” seems like such an obvious enterprise into which companies should channel their energies, perhaps a different mode of delivery is necessary to truly advance the cause and proactively safeguard the userbase.
Education about generative AI harms – and, frankly, any type of misuse or abuse that can occur – should be primarily conveyed or provided within the user’s in-platform experience, and ideally done in a seamless way. This may look like notifications, prompts, interstitials, loading screens (and pre-rolls), and creative methods like the earning of badges and other forms of gamification. Furthermore, the educational content has to be compelling, whether it is delivered by an influencer or celebrity, is highly relatable, and/or implicates an appropriate level of intrigue.
I have found that the best content takes advantage of the natural ways our attention is captured and forces us to pause and reflect, much like an “inciting incident” in a story compels us to keep reading). By and large, companies have done a mediocre job in accomplishing this. But here is another chance. There is much conversation about “media literacy” and “digital citizenship” and “civility” – all incredibly critical skills to cultivate. We just need to make sure they are strategically and compellingly taught to users across society. Ultimately, this should improve the kind of behavioral choices that are being made with these tools, consequently fostering healthier spaces online.
The Role of Users in Preventing Generative AI Abuse
You and I likely make hundreds of choices online every single day and it is easy to operate in a constantly semi-distracted manner as we type, click, multitask, and attempt to be productive. Unfortunately, this leads to a paucity of attention and intention with what we are doing. Coupled with an invincibility complex which may convince us that our chances of victimization are much smaller than others, it’s easy to be tricked, scammed, or attacked in a manner that could have been prevented. Generative AI can support and enhance our own efforts to stay safe online, and ideally will reduce the scope and prevalence of victimization that is happening more broadly.
Use Software to Detect AI Generated Images, Video, and Text
I discussed deepfakes, catfishing, and sextortion enabled by generative AI in my previous piece. Generally speaking, there are signals which can help a user identify when someone (or something) is not as it seems. It may be as simple as zooming into photos and videos to determine if they look airbrushed or otherwise abnormal. The lighting and shadows in the content – or the presence of certain artefacts – might also give it away as AI-generated. It’s fair, though, to point out that such practices are a lot easier on a laptop or desktop computer and very difficult on a mobile phone (which is the preferred device for most in technologically developed countries and often the only device for many in the majority world).
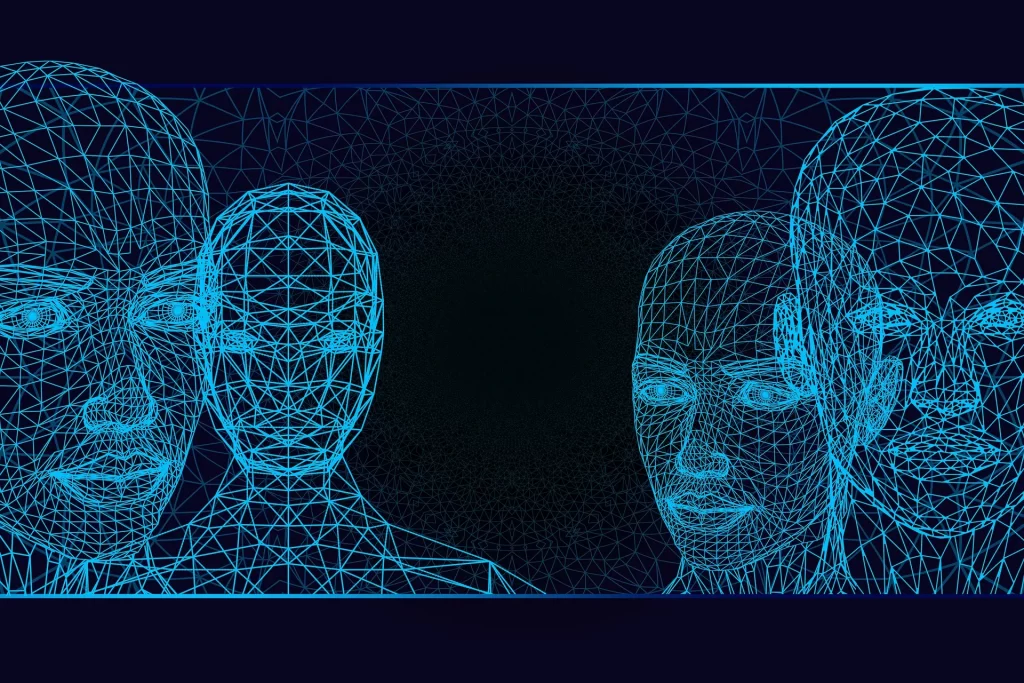
One can also use reverse image search engines to identify the provenance of certain content. If it is something seen in multiple locations across the Internet, it is likely a stock photo or an AI creation. Third-party software like Sensity, Fawrensian, Illuminarty, and FakeCatcher can also be employed to determine whether an image or video is synthetic. In time, my hope is that the features in these detection apps will be built in at the OS level on our devices – and a part of the user experience on our favorite online platforms – and flag or otherwise notify us that the content we’re looking at may be fake. Given my previous point about the challenges in identifying deepfakes on a mobile device, users across the globe would highly benefit from technological assistance from iOS and Android to accomplish this.
In my last writeup, I explained the use of conversational AI agents to earn trust, elicit romantic bonding, and invite intimate disclosures that can then be used to exploit a target. All users must evaluate the language utilized to determine whether they are communicating with a bot or another human. If a human user notices puzzling typos and changes in tone, repetitive phrasings, or a disconnect between the words used and the context in which they are presented, their spidey sense should tingle. They can also use software such as Open AI’s classifier, GPTZero, or GLTR, all of which are trained on large language models and can help identify AI-generated text. It is also highly advisable to verify the identity of an online romantic interest in real time via video chat. That said, our Center has dealt with cases where the target (usually a male) does get to video chat with the woman they’ve been romantically text chatting with, only to eventually realize that the woman has been covertly working with another person (usually a male) who then launches the threats, extortion attempts, and harm.
Enlist Users to Help Label Problematic AI Generated Content
We also need to enlist users in the very process and outcome of building systems that produce fairer and more accurate outputs. To that end, could there be a simple, frictionless way for users to help improve the quality of the LLMs that are producing new content? An assortment of tags available after receiving output from a prompt can allow them to label that which is (for example) “biased,” “hateful,” “sexual,” “violent,” “racist,” or “mature.” This can consequently help inform decisions on what content should be removed and what should be kept from the continually growing corpus of data. I realize this assumes that the labels applied will be fairly accurate and that this system won’t be gamed or abused at scale, but perhaps it is a starting point upon which to build. This may be especially true if the userbase has been encouraged to take more ownership of their personal online communities, channels, and comment threads.
Stay Aware, Skeptical, and Safeguard Yourself
One would hope that plenty of lessons would have been learned during the last two election cycles related to misinformation and fake news. One would hope that every Internet user viscerally understand that a growing amount of online content is not legitimate, objective, factual, or innocuous because of generative AI. Unfortunately, we may be giving ourselves too much credit and arguably should redouble our efforts to be mindful of what we consume. As I work with middle and high schoolers, my hope is that they increasingly take pride in being media literate and identifying deceptive content, instead of regularly and uncaringly being duped and shrugging it off with a “whoops LOL.” Skepticism is healthy. Users should, especially in these times, operate and interact with much wisdom and discretion.
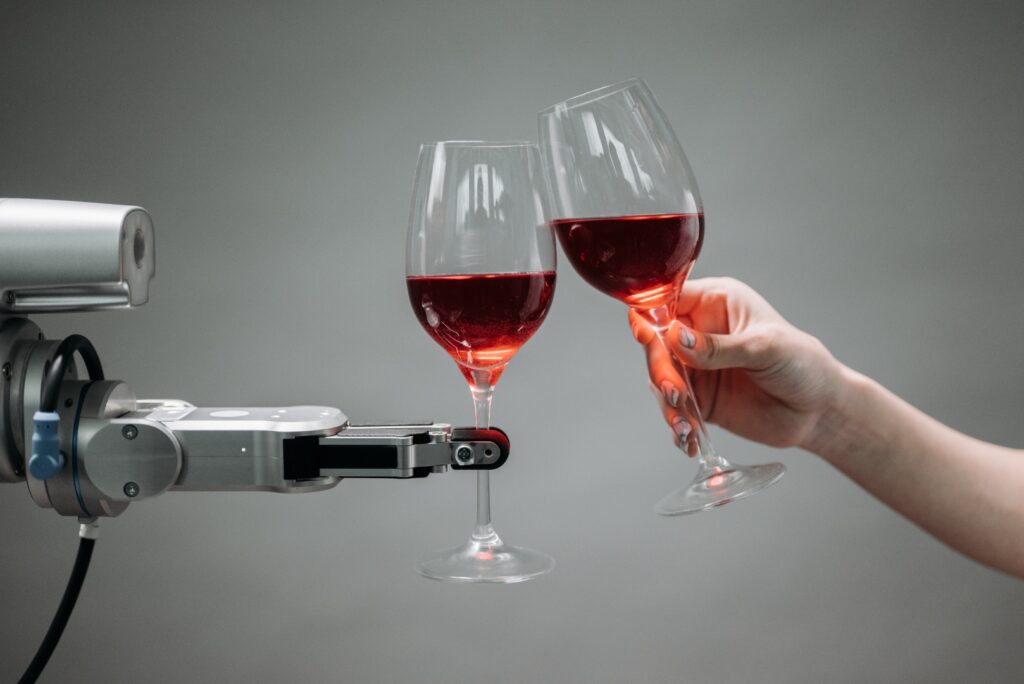
Relatedly, we recommend that users trust their gut. All of us have been interacting with other humans our entire life (even if mediated by technologies like FaceTime, Zoom, Google Meet, and WhatsApp). When it comes to determining the legitimacy of images, videos, or accounts, users should not dismiss what they think is amiss. They should trust their senses, their subconscious, and their established heuristics to notice visual, auditory, or linguistic inconsistencies and then – most importantly – act. They should not just move on and keep doing whatever they were doing.
At the same time, users should minimize their vulnerability to those intent on harassing, harming, or tricking them by ignoring messages and connection requests from unverified senders. They should also investigate the identity of certain content creators to ensure they are humans with honorable motives, and attempt to control what they (or others) have made publicly available about them (which then might be marshaled against them). Basically, putting into practice the principles of “Internet Safety 101” can go a long way in forestalling victimization.
While a variety of stakeholders can and will shape how generative AI impacts society, platforms and users are currently best positioned to protect against misuse and abuse in specific and tangible ways. Further research and evaluative studies through collaborations between scholars and practitioners will be essential to create new knowledge and derive the most benefit from this game-changing technology. It is my sincere hope, though, that we are both timely and proactive in our efforts given the sheer power and capacity of what we now have at our fingertips.
Featured image: Saweetie
Other images: Pavel Danilyuk, Sanket Mishra, Sujins, Geralt
The post Generative AI Risks and Harms – The Role of Platforms and Users appeared first on Cyberbullying Research Center.